Complexity Studies of Firm Dynamics
Abstract:
This thesis consists of three projects employing complexity economics methods to explore firm dynamics. The first is the Firm Ecosystem Model, which addresses the institutional conditions of capital access and entrenched competitive advantage. Larger firms will be more competitive than smaller firms due to efficiencies of scale, but the persistence of larger firms is also supported institutionally through mechanisms such as tax policy, capital access mechanisms and industry-favorable legislation. At the same time, evidence suggests that small firms innovate more than larger firms, and an aggressive firm-as-value perspective incentivizes early investment in new firms in an attempt to capture that value. The Ecological Firm Model explores the effects of the differences in innovation and investment patterns and persistence rates between large and small firms.
The second project is the Structural Inertia Model, which is intended to build theory around why larger firms may be less successful in capturing new marketshare than smaller firms, as well as to advance fitness landscape methods. The model explores the possibility that firms with larger scopes may be less effective in mitigating the costs of cooperation because conditions may arise that cause intrafirm conflicts. The model is implemented on structured fitness landscapes derived using the maximal order of interaction (NM) formulation and described using local optima networks (LONs), thus integrating these novel techniques.
Finally, firm dynamics can serve as a proxy for the ease at which people can voluntarily enter into the legal cooperative agreements that constitute firms. The third project, the Emergent Firm model, is an exploration of how this dynamic of voluntary association may be affected by differing capital institutions, and explores the macroeconomic implications of the economies that emerge out of the various resulting firm populations.
Parameter Estimation and Modeling of Visceral Leishmaniasis Transmission
Abstract:
The Visceral Leishmaniasis (VL) is primarily endemic in five countries, with India and Sudan having the highest burden. The risk factors associated with VL are either unknown in some regions or vary drastically among empirical studies. Here, a dynamical model, motivated and informed by field data from the literature, is analyzed and employed to identify and quantify the impact of region-dependent risks on the VL transmission dynamics. We constructed a parameter estimation scheme that was developed fusing model-derived quantities with empirical data. The dynamics of VL depends on the estimates of the control reproductive number, interpreted as the average number of secondary infections generated by a single infectious individual during the infectious period. The distribution was estimated for both India (with mean ) and Sudan (with mean). The parameter sensitivity analysis suggests that the average biting rate, transmission probabilities and the treatment rate are among the most sensitive parameters for both countries. The comparative assessment of VL transmission dynamics in both India and Sudan was carried out by parameter sensitivity analysis on VL prevalence level. The results identify that the treatment rate and clinical symptoms developmental rate are two highly sensitive parameters to VL prevalence. We found that the estimates of transmission probability are significantly different between India (from human to sandflies with mean; from sandflies to human with mean) and Sudan (from human to sandflies with mean; from sandflies to human with mean ). Our results have significant implications to elimination. An increasing focus on elimination requires a review of priorities within the VL control agenda. The development of systematic implementation of control programs based on identified risk factors (such as monitoring of asymptomatically infected individuals) has a strong transmission-blocking potential.
Abstract:
Network analysis is a key conceptual orientation and analytical tool in the social sciences that emphasizes the embeddedness of individual behavior within a larger web of social relations. The network approach is used to better understand the cause and consequence of social interactions which cannot be treated as independent. The relational nature of network data and models, however, amplify the methodological concerns associated with inaccurate or missing data. This dissertation addresses such concerns via three projects. As a motivating substantive example, Project 1 examines factors associated with the selection of interaction partners by students at a large urban high school implementing a reform which, like many organizational improvement initiatives, is associated with a theory of change that posits changes to the structuring of social interactions as a central causal pathway to improved outcomes. A distinctive aspect of the data used in Project 1 is that it was a complete egocentric network census – in addition to being asked about their own relationships, students were asked about the relationships between alters that they nominated in the self-report. This enables two unique examinations of methodological challenges in network survey data collection: Project 2 examines the factors related to how well survey respondents assess the strength of social connections between others, finding that “informant” competence corresponds positively with their social proximity to target dyad as well as their centrality in the network. Project 3 explores using such third-party reports to augment network imputation methods, and finds that incorporating third-party reports into model-based methods provides a significant boost in imputation accuracy. Together these findings provide important implications for collecting and extrapolating data in research contexts where a complete social network census is highly desirable but infeasible.
Intramyocellular Lipids and the Progression of Muscular Insulin Resistance
Abstract:
Diabetes is a disease characterized by reduced insulin action and secretion, leading to elevated blood glucose. In the 1990s, studies showed that intravenous injection of fatty acids led to a sharp negative response in insulin action that subsided hours after the injection. The molecule associated with diminished insulin signalling response was a byproduct of fatty acids, diacylglycerol. This dissertation is focused on the formulation of a model built around the known mechanisms of glucose and fatty acid storage and metabolism within myocytes, as well as downstream effects of diacylglycerol on insulin action. Data from euglycemic-hyperinsulinemic clamp with fatty acid infusion studies are used to validate the qualitative behavior of the model and estimate parameters. The model closely matches clinical data and suggests a new metric to determine quantitative measurements of insulin action downregulation. Analysis and numerical simulation of the long term, piecewise smooth system of ordinary differential equations demonstrates a discontinuous bifurcation implicating nutrient excess as a driver of muscular insulin resistance.
Mathematical and Computational Models of Cancer and The Immune System
Abstract:
The immune system plays a dual role during neoplastic progression. It can suppress tumor growth by eliminating cancer cells, and also promote neoplastic expansion by either selecting for tumor cells that are fitter to survive in an immunocompetent host or by establishing the right conditions within the tumor microenvironment. First, I present a model to study the dynamics of subclonal evolution of cancer. I model selection through time as an epistatic process. That is, the fitness change in a given cell is not simply additive, but depends on previous mutations. Our ansysis indicate that tumors are composed of myriads of small subclones at the time of diagnosis. Because some of these rare subclones harbor pre-existing treatment-resistant mutations, they present a major challenge to precision medicine. Second, I address the question of self and non-self discrimination by the immune system, which is fundamental in the field in cancer immunology. By performing a quantitative analysis of the biochemical properties of thousands of MHC class I peptides, I find that hydrophobicity of T cell receptors contact residues is a hallmark of immunogenic epitopes. Based on these findings, I further develop a computational model to predict immunogenic epitopes which facilitate the development of T cell vaccines against pathogen and tumor antigens. Third, I study the evolutionary and immunological basis for clinical response to immune checkpoint blockade therapy using melanoma and lung genomic data from clinical responders and non-responders to CTLA-4 blockade and PD-1 blockade, respectively. For this purpose, I develop a mathematical model to infer the probability of long-term clinical response. By fitting my mathematical model to these genomic data sets, I am able to calculate the probability of immunogenic neoepitopes per neopeptide. Moreover, I find that at least one immunogenic neoepitope must be present in a tumor in order lead to clinical response. I further find extensive neoepitope depletion in tumors of some non-responders. This result suggests that these tumors have undergone "immunoediting," thus impacting clinical response to immune checkpoint blockade therapy. Lastly, I study the effect of early detection in the context of Ebola. I develope a simple mathematical model calibrated to the transmission dynamics of Ebola virus in West Africa. My findings suggest that a strategy that focuses on early diagnosis of high-risk individuals, caregivers, and health-care workers at the pre-symptomatic stage, when combined with public health measures to improve the speed and efficacy of isolation of infectious individuals, can lead to rapid reductions in Ebola transmission.
Solvable Time-Dependent Models in Quantum Mechanics
ABSTRACT
In the traditional setting of quantum mechanics, the Hamiltonian operator does not depend on time. While some Schrödinger equations with time-dependent Hamiltonians have been solved, explicitly solvable cases are typically scarce. This thesis is a collection of papers in which this first author along with Suslov, Suazo, and Lopez, has worked on solving a series of Schrödinger equations with a time-dependent quadratic Hamiltonian that has applications in problems of quantum electrodynamics, lasers, quantum devices such as quantum dots, and external varying fields.
In particular the author discusses a new completely integrable case of the time-dependent Schrödinger equation in Rn with variable coefficients for a modified oscillator, which is dual with respect to the time inversion to a model of the quantum oscillator considered by Meiler, Cordero-Soto, and Suslov. A second pair of dual Hamiltonians is found in the momentum representation. Our examples show that in mathematical physics and quantum mechanics a change in the direction of time may require a total change of the system dynamics in order to return the system back to its original quantum state.
The author also considers several models of the damped oscillators in nonrelativistic quantum mechanics in a framework of a general approach to the dynamics of the timedependent Schrödinger equation with variable quadratic Hamiltonians. The Green functions are explicitly found in terms of elementary functions and the corresponding gauge transformations are discussed. The factorization technique is applied to the case of a shifted harmonic oscillator. The time-evolution of the expectation values of the energy related operators is determined for two models of the quantum damped oscillators under consideration. The classical equations of motion for the damped oscillations are derived for the corresponding expectation values of the position operator.
Finally, the author constructs integrals of motion for several models of the quantum damped oscillators in a framework of a general approach to the time-dependent Schrödinger equation with variable quadratic Hamiltonians. An extension of the Lewis–Riesenfeld dynamical invariant is given. The time-evolution of the expectation values of the energy related positive operators is determined for the oscillators under consideration. A proof of uniqueness of the corresponding Cauchy initial value problem is discussed as an application.
Epidemic Dynamics of Metapopulation Models
ABSTRACT
Mathematical modeling of infectious diseases can help public health officials to make decisions related to the mitigation of epidemic outbreaks. However, over or under estimations of the morbidity of any infectious disease can be problematic. Therefore, public health officials can always make use of better models to study the potential implication of their decisions and strategies prior to their implementation. Previous work focuses on the mechanisms underlying the different epidemic waves observed in Mexico during the novel swine origin influenza H1N1 pandemic of 2009 and showed extensions of classical models in epidemiology by adding temporal variations in different parameters that are likely to change during the time course of an epidemic, such as, the influence of media, social distancing, school closures, and how vaccination policies may affect different aspects of the dynamics of an epidemic. This current work further examines the influence of different factors considering the randomness of events by adding stochastic processes to meta-population models. I present three different approaches to compare different stochastic methods by considering discrete and continuous time. For the continuous time stochastic modeling approach I consider the continuous-time Markov chain process using forward Kolmogorov equations, for the discrete time stochastic modeling I consider stochastic differential equations using Wiener's increment and Poisson point increments, and also I consider the discrete-time Markov chain process. These first two stochastic modeling approaches will be presented in a one city and two city epidemic models using, as a base, our deterministic model. The last one will be discussed briefly on a one city SIS and SIR-type model.
Diffusive Instability and Aggregation in Epidemics
ABSTRACT
Mathematical epidemiology helps formalize and quantify epidemiological processes such as disease outbreak, epidemic recurrence, disease invasion and disease persistence in landscapes with well defined levels of heterogeneity. It is within the context of a mathematical framework that theoreticians address “what if” questions that are central to the development of public health policy or that aid the understanding of disease evolution. Two kinds of modeling frameworks have been proposed in the context of spatial epidemics. They are reaction diffusion and spatial contact models. The first class of models assumes that individuals diffuse at random while the second assumes that individuals “disperse” via distribution kernels. This dissertation attempts to tease out some of the reasons behind the theoretical predictions generated by both classes of models in the context of disease dynamics.
Spatial models quantify disease spread in terms of epidemiological parameters (infection and recovery rates) that influence the speed of disease propagation -traveling epidemic fronts. A recurrent assumption behind both type of models is uniformity in disease propagation. Such an assumption while unrealistic facilitates the mathematical analysis. In this dissertation the assumption of uniform mixing (homogeneity) is relaxed, spatial heterogeneity in the transmission process is allowed.
A novel reaction diffusion model is introduced and used to identify necessary and sufficient conditions for the aggregation of individuals that may result in response to the introduction of a communicable disease. The methodology and techniques used in the analysis of this model, which exhibits diffusive instability, include Turing theory, which as far as I know, has not been used in this context.
The mathematical results are obtained in a framework that ignores demo- graphic change. Extensions incorporate demographic factors making the mathematical analysis beyond my means. Hence I explore the impact of demography numerically. The analytical results together with the numerical simulations suggest that the incorporation of different diffusion coefficients for at least two distinct infectious classes are not only necessary for diffusive instability but also serve to reduce the average number of contacts between infected and susceptible individuals which lead to reductions in prevalence.
Consequences of short-term mobility across heterogeneous risk environments: the 2014 West African Ebola outbreak.
Abstract:
In this dissertation, the potential impact of some social, cultural and economic factors on the Ebola Virus Disease (EVD) dynamics and control are studied. In Chapter two, the inability to detect and isolate a large fraction of EVD-infected individuals before symptoms onset is addressed. A mathematical model, calibrated with data from the 2014 West African outbreak, is used to show the dynamics of EVD control under various quarantine and isolation effectiveness regimes. It is shown that in order to make a difference it must reach a high proportion of the infected population. The effect of EVD-dead bodies has been incorporated in the quarantine effectiveness. In Chapter four, the potential impact of differential risk is assessed. A two-patch model without explicitly incorporate quarantine is used to assess the impact of mobility among communities at risk of EVD. It is shown that the overall EVD burden may lessen when mobility in this artificial high-low risk society is allowed. The cost that individuals in the low-risk patch must pay, as measured by secondary cases is highlighted. In Chapter five a model explicitly incorporating patch-specific quarantine levels is used to show that quarantine a large enough proportion of the population under effective isolation leads to a measurable reduction of secondary cases in the presence of mobility. It is shown that sharing limited resources can improve the effectiveness of EVD effective control in the two-patch high-low risk system. Identifying the conditions under which the low-risk community would be willing to accept the increases in EVD risk, needed to reduce the total number of secondary cases in a community composed of two patches with highly differentiated risks, has not been addressed. In summary, this dissertation looks at EVD dynamics within an idealized highly polarized world where resources are primarily in the hands of a low-risk community -- a community of lower density, higher levels of education and reasonable health services -- that shares a ``border'' with a high-risk community that lacks minimal resources to survive an EVD outbreak.
Characterization of the Mathematical Theoretical Biology Institute as a Vygotskian-Holzman Zone of Proximal Development
ABSTRACT.
The Mathematical and Theoretical Biology Institute (MTBI) is a summer research program for undergraduate students, largely from underrepresented minority groups. Founded in 1996, it serves as a 'life-long' mentorship program, providing continuous support for its students and alumni. This study investigates how MTBI supports student development in applied mathematical research. This includes identifying of motivational factors to pursue and develop capacity to complete higher education.
The theoretical lens of developmental psychologists Lev Vygotsky (1978, 1987) and Lois Holzman (2010) that sees learning and development as a social process is used. From this view student development in MTBI is attributed to the collaborative and creative way students co-create the process of becoming scientists. This results in building a continuing network of academic and professional relationships among peers and mentors, in which around three quarters of MTBI PhD graduates come from underrepresented groups.
The extent to which MTBI creates a Vygotskian learning environment is explored from the perspectives of participants who earned doctoral degrees. Previously hypothesized factors (Castillo-Garsow, Castillo-Chavez and Woodley, 2013) that affect participants’ educational and professional development are expanded on.
Factors identified by participants are a passion for the mathematical sciences; desire to grow; enriching collaborative and peer-like interactions; and discovering career options. The self-recognition that they had the ability to be successful, key element of the Vygotskian-Holzman theoretical framework, was a commonly identified theme for their educational development and professional growth.
Participants characterize the collaborative and creative aspects of MTBI. They reported that collaborative dynamics with peers were strengthened as they co-created a learning environment that facilitated and accelerated their understanding of the mathematics needed to address their research. The dynamics of collaboration allowed them to complete complex homework assignments, and helped them formulate and complete their projects. Participants identified the creative environments of their research projects as where creativity emerged in the dynamics of the program.
These data-driven findings characterize for the first time a summer program in the mathematical sciences as a Vygotskian-Holzman environment, that is, a `place’ where participants are seen as capable applied mathematicians, where the dynamics of collaboration and creativity are fundamental components.
Multiscale Modeling of Cancer
ABSTRACT
The progression of cancer has been identified as a microcosm of Darwinian evolution. Although the concept of ”somatic evolution” is well accepted, one can only correlate the genetic mutations that mark a cancer to the malignant cell phenotypes that cause it. It is often implicated that certain gene abnormalities cause the malignant cell phenotype by rewiring the flow of subcellular signal transduction of extracellular chemicals; however, correlating a set of genetic mutations to a specific pathway and cellular function, also called gene ontology, is not enough to implicate that pathway as the cause of malignant progression. The goal of this research is to provide a multiscale quantitative framework for evaluating whether genetic mutations might lead to tumor progression. Colon cancer and the adenomatosis polyposis coli (APC) mutation are used as the main example of how to construct this framework because there is a copious body of research about how the mutation affects malignant progression. Specifically, the hypothesis that is validated is that the specific mutations that lower the binding rate of APC to its downstream targets are selected for to alter the proliferation, apoptosis, and differentiation rates of individual cells to maximize individual cell survival and as a result increases the maximum sustainable colon crypt size. A stochastic model was developed of the gene network that describes the signal transduction of wingless homolog (WNT), an extracellular factor important for colon stem cell division, and it is shown that the APC mutation can mimic the fold changes in the expression of cell cycle and differentiation phenotype encoding genes. Based on these observations, a multiscale model was approximated with an ordinary differential equation system and it was shown that the malignant phenotype encoded by the APC mutation is able to survive as long as the phenotype it encodes reduces the dependence on extracellular WNT enough to satisfy an inequality involving the growth and DNA repair rate of colon stem cells.
Applying Distributional Approaches to Understand Patterns of Urban Differentiation
ABSTRACT
Urban scaling analysis has introduced a new scientific paradigm to the study of cities. With it, the notions of size, heterogeneity and structure have taken a leading role. These notions are assumed to be behind the causes for why cities differ from one another, sometimes wildly. However, the mechanisms by which size, heterogeneity and structure shape urban economic output are still unclear, and empirical studies that measure them and their consequences are still inconclusive. Given the rapid rate of urbanization around the globe, we need precise and formal mathematical understandings of these matters. In this context, I perform in this dissertation probabilistic, distributional and computational explorations of (i) how the broadness, or narrowness, of the distribution of individual productivities within cities determines what and how we measure urban systemic output, (ii) how urban scaling may be expressed as a statistical statement when urban metrics display strong stochasticity, (iii) how the process of aggregation constrain the variability of total urban output, and (iv) how the structure of urban skills diversification within cities induces a multiplicative process in the production of urban output.
Quantitative Modeling Methods for Analyzing Clinical to Public Health Problems
ABSTRACT
Statistical Methods have been widely used in understanding factors for clinical and public health data. Statistical hypotheses are procedures for testing pre-stated hypotheses. The development and properties of these procedures as well as their performance are based upon certain assumptions. Desirable properties of statistical tests are to maintain validity and to perform well even if these assumptions are not met. A statistical test that maintains such desirable properties is called robust. Mathematical models are typically mechanistic framework, used to study dynamic interactions between components (mechanisms) of a system, and how these interactions give rise to the changes in behavior of the system as a whole over time. Most mathematical models require input factors that consist of describing mechanisms as a functions of parameters and initial conditions for both independent and dependent model relevant quantities (variables). These are not always known with sufficient degree of certainty because of natural variation, error in measurements, or lack of current techniques to measure them. In this thesis, I have developed a study that uses novel techniques to link statistical and mathematical modeling methods guided by limited data from developed and developing regions in order to address pressing clinical questions of interest via statistical and mathematical methods that incorporate uncertainty analysis in order to quantify the degree of confidence in the parameter estimates.
Many times there are challenges in collecting sufficient data including lack of financial and human resources or difficulty in meeting ethical standards. The techniques provided are multi-step procedures to systematically amalgamate mathematical and statistical methods to achieve goals and are divided in my dissertation into three parts. The first part of the study focuses on designing, implementing, collecting, cleaning, and summarizing empirical data from survey using various simple statistical methods. As an example to the procedure, we gather and analyze the only national survey of hospitals ever conducted regarding patient controlled analgesia (PCA) practices among 168 hospitals across 40 states, in order to assess risks before putting patients on PCA. In the second part of the study, we quantified uncertainty associated with the collection of data that are often limited. This was carried out via sensitivity analysis on data from James A Lovell Federal Healthcare Center. The data included certain characteristics of patients treated at this center and was used to evaluate the effect of Benign Prostatic Hypertrophy (BPH) on sleep architecture in patients with Obstructive Sleep Apnea (OSA) diagnosed by polysomnography. The analysis of this data set resulted in addressing some additional theoretical questions such as (1) how to estimate the distribution of a variable in order to retest hypothesis, and (2) how changes on assumptions (like monotonicity, nonlinearity, etc) related to the influence of the effect of the independent variable on the outcome variable, when studying associations. To address these questions we use multiple techniques such as Partial Rank Correlation Coefficients (PRCC) based sensitivity analysis, Fractional Polynomials and statistical models. In the third part of the study, the goal was to link results from the statistical model to provide more reasonable mathematical modeling framework. As an example for this goal, we primarily studied the role of age-specific susceptibility on the dynamics of Visceral Leishmaniasis (VL) in the Indian state of Bihar. In this part, statistical results provided ideas on the choice of the mathematical modeling framework and estimates of its parameters.
Niche Construction, Sustainability and Evolutionary Ecology of Cancer
ABSTRACT
In complex consumer-resource type systems, where diverse individuals are interconnected and interdependent, one can often anticipate what has become known as the tragedy of the commons, i.e., a situation,when overly efficient consumers exhaust the common resource, causing collapse of the entire population. In this dissertation I use mathematical modeling to explore different variations on the consumer-resource type systems, identifying some possible transitional regimes that can precede the tragedy of the commons. I then reformulate it as a game of a multi-player prisoner's dilemma and study two possible approaches for preventing it, namely direct modification of players' payoffs through punishment/reward and modification of the environment in which the interactions occur.
I also investigate the questions of whether the strategy of resource allocation for reproduction or competition would yield higher fitness in an evolving consumer-resource type system and demonstrate that the direction in which the system will evolve will depend not only on the state of the environment but largely on the initial composition of the population. I then apply the developed framework to modeling cancer as an evolving ecological system and draw conclusions about some alternative approaches to cancer treatment.
Lifelong Adaptive Neuronal Learning for Autonomous Multi-Robot Demining in Colombia, and Enhancing the Science, Technology and Innovation Capacity of the Ejército Nacional de Colombia
ABSTRACT
After the recent end of a 52-year guerrilla war in Colombia, the country is engaged in a large-scale, humanitarian demining effort. However, the vast areas to be demined, the extreme terrains and shortage of demining personnel and mine detection dogs are hampering this effort. This motivates the development of autonomous robots for humanitarian demining. To achieve this goal, we need to address two key problems. First, we need to develop a robotic controller that plans a path to cover a search area as completely and efficiently as possible, i.e. the classic, coverage path planning (CPP) problem. Second, we need to enhance the science, technology and innovation (STI) capacity of the Ejército Nacional de Colombia (the Colombian National Army).
To address the robotic controller problem, we apply Thangavelautham's (2012, 2017) Artificial Neural Tissue (ANT) control algorithm to solve the CPP task for demining. Trained by a simple genetic algorithm, ANT is an artificial neural network (ANN) controller with a sparse, coarse coding network architecture and adaptive activation functions. Starting from the exterior boundary of an open grid area, computer simulations of an ANT multi-robot team can cover a square area completely in linear time, and circular area almost completely in quasi-linear time, where time complexity is measured by the number of grid cells to be covered. ANT achieves these results by maximizing a single global fitness function of the number of newly visited grid cells minus a penalty on the number of revisited grid cells.
However, ANNs have a fundamental deficiency of catastrophic forgetting, where neural network connection weights trained for one task do not work for an alternative task, and new connection weights must be learned to solve a new task. This hinders ANN controllers from operating effectively in dynamic environments. We present a proof of concept on how ANT can mitigate catastrophic forgetting. Specifically, we show that ANT neural network weights and the number neurons comprising a solution for one CPP task (e.g. covering a square area) can be frozen, and by re-training only the ANT activation function and output behavior parameters to solve an alternative CPP task (e.g. covering a circular area), this new solution is comparable to training all the ANT network weights and parameters ab initio. We call this focus on training the ANT activation function parameters, Lifelong Adaptive Neuronal Learning (LANL), where the number of neurons and connection weights remain substantially unchanged in certain problem domains, and the algorithm can learn different activation function parameters to solve different domain subsets, and thereby, engage in lifelong learning. We hope that LANL will allow us to build a library of activation function parameters that ANT can recall for different and dynamic CPP scenarios.
Even if we solve the robotic controller problem, the Colombian National Army (COLAR) will need capable STI personnel to modify robots, develop new robots and address other demining problems, such as explosive detection. Given the dearth of STI capacity in Colombia, we propose to develop an elite, COLAR science and technology officer corps, ÉLITE (Equipo de Líderes en Investigación y Tecnología del Ejército). ÉLITE is inspired by the Israel Defense Forces science and technology program, Talpiot.
Energy use scaling and alarm spread in social ants: An investigation using multi-agent simulation and object tracking
ABSTRACT
Social insect groups, such as bees, termites, and ants, allow us to study how group-level behaviors emerge from the aggregated actions and interactions of individuals. Ants have the unique advantage that whole colonies can be observed in artificial, laboratory nests, and each individual's behavior can be continuously tracked using imaging software. In this dissertation, I study two group behaviors: (1) the spread of alarm signals from three agitated ants to a group of 61 quiescent nestmates, and (2) the reduction in per-capita energy use as colonies scale in size from tens of ants to thousands. For my first experiment, I track the motion of Pogonomyrmex californicus ants using an overhead camera, and I analyze how propagation of an initial alarm stimulus affects their walking speeds. I then build an agent-based model that simulates two-dimensional ant motion and the spread of the alarmed state. I find that implementing a simple set of rules for motion and alarm signal transmission reproduces the empirically observed speed dynamics. For the second experiment, I simulate social insect colony workers that collectively complete a set of tasks. By assuming that task switching is energetically costly, my model recovers a metabolic rate scaling pattern, known as hypometric metabolic scaling. This relationship, which predicts an organism’s metabolic rate from its mass, is observed across a diverse set of social insect groups and animal species. The results suggest an explicit link between the degree of workers’ task specialization and whole-colony energy use.
Integrability of Quadratic Non-autonomous Quantum Linear Systems
ABSTRACT
The Quantum Harmonic Oscillator is one of the most important models in Quantum Mechanics. Analogous to the classical mass vibrating back and forth on a spring, the quantum oscillator system has attracted substantial attention over the years because of its importance in many advanced and difficult quantum problems. This thesis deals with solving generalized models of the time-dependent Schroedinger equation which are called generalized quantum harmonic oscillators, and these are characterized by an arbitrary quadratic Hamiltonian of linear momentum and position operators. The primary challenge in this work is that most quantum models with time-dependence are not solvable explicitly, yet this challenge became the driving motivation for this work.
In this thesis, the methods used to solve the time-dependent Schroedinger equation are the fundamental singularity (or Green’s function) and the Fourier (eigenfunction expansion) methods. Certain Riccati- and Ermakov-type systems arise, and these systems are highlighted and investigated. The overall aims of this thesis are to show that quadratic Hamiltonian systems are completely integrable systems, and to provide explicit approaches to solving the time-dependent Schroedinger equation governed by an arbitrary quadratic Hamiltonian operator. The methods and results established in the thesis are not yet well recognized in the literature, yet hold for high promise for further future research.
Finally, the most recent results in the thesis correspond to the harmonic oscillator group and its symmetries. A simple derivation of the maximum kinematical invariance groups of the free particle and quantum harmonic oscillator is constructed from the view point of the Riccati- and Ermakov-type systems, which shows an alternative to the traditional Lie Algebra approach. To conclude, a missing class of solutions of the time-dependent Schroedinger equation for the simple harmonic oscillator in one dimension is constructed. Probability distributions of the particle linear position andmomentum, are emphasized with Mathematica animations. The eigenfunctions qualitatively differ from the traditional standing waves of the one-dimensional Schroedinger equation. The physical relevance of these dynamic states is still questionable, and in order to investigate their physical meaning, animations could also be created for the coherent states. This might be addressed in future work.
A Neuronal Network Model of Drosophila Antennal Lobe
ABSTRACT
Olfaction is an important sensory modality for behavior since odors inform animals of the presence of food, potential mates, and predators. The fruit fly, Drosophila melanogaster, is a favorable model organism for the investigation of the biophysical mechanisms that contribute to olfaction because its olfactory system is anatomically similar to but simpler than that of vertebrates. In the Drosophila olfactory system, sensory transduction takes place in olfactory receptor neurons housed in the antennae and maxillary palps on the front of the head. The first stage of olfactory processing resides in the antennal lobe, where the structural unit is the glomerulus. There are at least three classes of neurons in the antennal lobe - excitatory projection neurons, excitatory local neurons, and inhibitory local neurons. The arborizations of the local neurons are confined to the antennal lobe, and output from the antennal lobe is carried by projection neurons to higher regions of the brain. Different views exist of how circuits of the Drosophila antennal lobe translate input from the olfactory receptor neurons into projection neuron output. We construct a conductance based neuronal network model of the Drosophila antennal lobe with the aim of understanding possible mechanisms within the antennal lobe that account for the variety of projection neuron activity observed in experimental data. We explore possible outputs obtained from olfactory receptor neuron input that mimic experimental recordings under different connectivity paradigms. First, we develop realistic minimal cell models for the excitatory local neurons, inhibitory local neurons, and projections neurons based on experimental data for Drosophila channel kinetics, and explore the firing characteristics and mathematical structure of these models. We then investigate possible interglomerular and intraglomerular connectivity patterns in the Drosophila antennal lobe, where olfactory receptor neuron input to the antennal lobe is modeled with Poisson spike trains, and synaptic connections within the antennal lobe are mediated by chemical synapses and gap junctions as described in the Drosophila antennal lobe literature. Our simulation results show that inhibitory local neurons spread inhibition among all glomeruli, where projection neuron responses are decreased relatively uniformly for connections of synaptic strengths that are homogeneous. Also, in the case of homogeneous excitatory synaptic connections, the excitatory local neuron network facilitates odor detection in the presence of weak stimuli. Excitatory local neurons can spread excitation from projection neurons that receive more input from olfactory receptor neurons to projection neurons that receive less input from olfactory receptor neurons. For the parameter values for the network models associated with these results, eLNs decrease the ability of the network to discriminate among single odors.
Potential Games and Competition in the Supply of Natural Resources
ABSTRACT
This dissertation discusses the Cournot competition and competitions in the exploitation of common pool resources and its extension to the tragedy of the commons. I address these models by using potential games and inquire how these models reflect the real competitions for provisions of environmental resources. The Cournot models are dependent upon how many firms there are so that the resultant Cournot-Nash equilibrium is dependent upon the number of firms in oligopoly. But many studies do not take into account how the resultant Cournot-Nash equilibrium is sensitive to the change of the number of firms. Potential games can find out the outcome when the number of firms changes in addition to providing the "traditional" Cournot-Nash equilibrium when the number of firms is fixed. Hence, I use potential games to fill the gaps that exist in the studies of competitions in oligopoly and common pool resources and extend our knowledge in these topics. In specific, one of the rational conclusions from the Cournot model is that a firm's best policy is to split into separate firms. In real life, we usually witness the other way around; i.e., several firms attempt to merge and enjoy the monopoly profit by restricting the amount of output and raising the price. I aim to solve this conundrum by using potential games. I also clarify, within the Cournot competition model, how regulatory intervention in the management of environmental pollution externalities affects the equilibrium number of polluters. In addition, the tragedy of the commons is the term widely used to describe the overexploitation of open-access common-pool resources. Open-access encourages potential resource users to continue to enter the resource up to the point where rents are exhausted. The resulting level of resource use is higher than is socially optimal, and in extreme cases can lead to the collapse of the resource and the communities that may depend on it. In this paper I use the concept of potential games to evaluate the relation between the cost of resource use and the equilibrium number of resource users in open access regimes. I find that costs of access and costs of production are sufficient to determine the equilibrium number of resource users, and that there is in fact a continuum between Cournot competition and the tragedy of the commons. I note that the various common pool resource management regimes identified in the empirical literature are associated with particular cost structures, and hence that this may be the mechanism that determines the number of resource users accessing the resource.
Patterns in Knowledge Production
Abstract:
This dissertation will look at large scale collaboration through the lens of online communities to answer questions about what makes a collaboration persist. Results address how collaborations attract contributions, behaviors that could give rise to patterns seen in the data, and the properties of collaborations that drive those behaviors. It is understood that collaborations, online and otherwise, must retain users to remain productive. However, before users can be retained they must be recruited. In the first project, a few necessary properties of the “attraction” function are identified by constraining the dynamics of an ODE (Ordinary Differential Equation) model. Additionally, more than 100 communities of the Stack Exchange networks are parameterized and their distributions reported. Collaborations do not exist in a vacuum, they compete with and share users with other collaborations. To address this, the second project focuses on an agent-based model (ABM) of a community of online collaborations using a mechanistic approach. The ABM is compared to data obtained from the Stack Exchange network and produces similar distributional patterns. The third project is a thorough sensitivity analysis of the model created in the second project. A variance based sensitivity analysis is performed to evaluate the relative importance of 21 parameters of the model. Results indicate that population parameters impact many outcome metrics, though even those parameters that tend towards a low impact can be crucial for some outcomes.
Prey-predator “Host-parasite” Models with Adaptive Dispersal: Application to Social Animals
Abstract:
Foraging strategies in social animals are often shaped by change in an organism’s natural surrounding. Foraging behavior can hence be highly plastic, time, and condition de-pendent. The motivation of my research is to explore the effects of dispersal behavior in predators or parasites on population dynamics in heterogeneous environments by developing varied models in different contexts through closely working with ecologists. My models include Ordinary Differential Equation (ODE)-type meta population models and Delay Differential Equation (DDE) models with validation through data. I applied dynamical theory and bifurcation theory with carefully designed numerical simulations to have a better understanding on the profitability and cost of an adaptive dispersal in organisms. My work on the prey-predator models provide important insights on how different dispersal strategies may have different impacts on the spatial patterns and also shows that the change of dispersal strategy in organisms may have stabilizing or destabilizing effects leading to extinction or coexistence of species. I also develop models for honeybee population dynamics and its interaction with the parasitic Varroa mite. At first, I investigate the effect of dispersal on honeybee colonies under infestation by the Varroa mites. I then provide an-other single patch model by considering a stage structure time delay system from brood to adult honeybee. Through a close collaboration with a biologist, a honeybee and mite population data was first used to validate my model and I estimated certain unknown parameters by utilizing least square Monte Carlo method. My analytical, bifurcations, sensitivity analysis, and numerical studies first reveal the dynamical outcomes of migration. In addition, the results point us in the direction of the most sensitive life history parameters affecting the population size of a colony. These results provide novel insights on the effects of foraging and Varroa mites on colony survival.
The Influence of Class Nonlinear Dynamics and Education on Socio-Economic Mobility
Abstract:
The dissertation addresses questions tied in to the challenges posed by the impact of environmental factors on the nonlinear dynamics of social upward mobility. The proportion of educated individuals from various socio-economic backgrounds is used as a proxy for the environmental impact on the status quo state.
Chapter 1 carries out a review of the mobility models found in the literature and sets the economic context of this dissertation. Chapter 2 explores a simple model that considers poor and rich classes and the impact that educational success may have on altering mobility patterns. The role of the environment is modeled through the use of a modified version of the invasion/extinction model of Richard Levins. Chapter 3 expands the socio-economic classes to include a large middle class to study the role of social mobility in the presence of higher heterogeneity. Chapter 4 includes demographic growth and explores what would be the time scales needed to accelerate mobility. The dissertation asked how long it will take to increase by 22% the proportion of educated from the poor classes under demographic versus non-demographic growth conditions. Chapter 5 summarizes results and includes a discussion of results. It also explores ways of modeling the influence of nonlinear dynamics of mobility, via exogenous factors. Finally, Chapter 6 presents economic perspectives about the role of environmental influence on college success. The framework can be used to incorporate the impact of economic factors and social changes, such as unemployment, or gap between the haves and have nots. The dissertation shows that peer influence (poor influencing the poor) has a larger effect than class influence (rich influencing the poor). Additionally, more heterogeneity may ease mobility of groups but results depend on initial conditions. Finally, average well-being of the community and income disparities may improve over time. Finally, population growth may extend time scales needed to achieve a specific goal of educated poor.
Robustness of Contact and Age- Aggregation in Influenza Models
ABSTRACT
The 2009-10 influenza and the 2014-15 Ebola pandemics brought once again urgency to an old question: What are the limits on prediction and what can we propose that is useful in the face of an epidemic outbreak?
This thesis looks first at the impact of limited access to vaccine stockpiles may have on single influenza outbreaks. We use this case study not only to highlight the challenges faced by populations that had limited access to vaccines stockpiles but also to identify the importance of exploring the same questions in more realistic settings.
It is well known that age-specific per capita constraint rates play an important role on the dynamics of communicable diseases and, influenza is, of course, no exception. And so, the challenges associated with measuring age-specific contact rates have not deterred efforts aimed at estimating them.
This thesis attempts to connect contact theory with age-specific contact data in the context of influenza outbreaks. Typically proportionate mixing has been used as the preferred theoretical mixing structure and we start from this model. In this context, the questions that drive the rest of this dissertation, in the context of influenza dynamics and control, are:
- What is the role of age-aggregation on the dynamics of a single outbreak? Or simply speaking, does the number of age-classes used to model a population make a significant difference on quantitative predictions?
- What would the age-specific optimal influenza vaccination policies be? Or, what are the age-specific vaccination policies needed to control an outbreak in the presence of limited or unlimited vaccine stockpiles?
Intertwined with the above questions are issues of resilience and uncertainty including, whether or not data collected on mixing (by social scientists) can be used effectively to address both questions in the context of influenza. Using influenza plausible scenarios, we proceed to “answer” both questions by assessing the role of aggregation (number and length of age classes) and robustness (does the aggregation scheme selected makes a difference) on influenza dynamics and control.
Applications of Nonlinear Systems of Ordinary Differential Equations and Volterra Integral Equations to Infectious Disease Epidemiology
ABSTRACT
In the field of infectious disease epidemiology, the assessment of model robustness outcomes plays a significant role in the identification, reformulation, and evaluation of preparedness strategies aimed at limiting the impact of catastrophic events (pandemics or the deliberate release of biological agents) or used in the management of disease prevention strategies, or employed in the identification and evaluation of control or mitigation measures. The research work in this dissertation focuses on: The comparison and assessment of the role of exponentially distributed waiting times versus the use of generalized non-exponential parametric distributed waiting times of infectious periods on the quantitative and qualitative outcomes generated by Susceptible-Infectious-Removed (SIR) models. Specifically, Gamma distributed infectious periods are considered in the three research projects developed following the applications found in [Bailey 1964, Anderson 1980, Wearing 2005, Feng 2007, Yan 2008, lloyd 2009, Vergu 2010]. i) The first project focuses on the influence of input model parameters, such as the transmission rate, mean and variance of Gamma distributed infectious periods, on disease prevalence, the peak epidemic size and its timing, final epidemic size, epidemic duration and basic reproduction number. Global uncertainty and sensitivity analyses are carried out using a deterministic Susceptible-Infectious-Recovered (SIR) model. The quantitative effect and qualitative relation between input model parameters and outcome variables are established using Latin Hypercube Sampling (LHS) and Partial rank correlation coefficient (PRCC) and Spearman rank correlation coefficient (RCC) sensitivity indices. We learnt that: For relatively low (R0 close to one) to high (mean of R0 equals 15) transmissibility, the variance of the Gamma distribution for the infectious period, input parameter of the deterministic age-of-infection SIR model, is key (statistically significant) on the predictability of the epidemiological variables such as the epidemic duration and the peak size and timing of the prevalence of infectious individuals and therefore, for the predictability these variables, it is preferable to utilize a nonlinear system of Volterra integral equations, rather than a nonlinear system of ordinary differential equations. The predictability of epidemiological variables such as the final epidemic size and the basic reproduction number are unaffected by (or independent of) the variance of the Gamma distribution for the infectious period and therefore for the choice on which type of nonlinear system for the description of the SIR model (VIE's or ODE's) is irrelevant. Although, for practical proposes, with the aim of lowering the complexity and number operations in the numerical methods, a nonlinear system of ordinary differential equations is preferred. The main contribution lies in the development of a model based decision-tool that helps determine when SIR models given in terms of Volterra integral equations are equivalent or better suited than SIR models that only consider exponentially distributed infectious periods. ii) The second project addresses the question of whether or not there is sufficient evidence to conclude that two empirical distributions for a single epidemiological outcome, one generated using a stochastic SIR model under exponentially distributed infectious periods and the other under the non-exponentially distributed infectious period, are statistically dissimilar. The stochastic formulations are modeled via a continuous time Markov chain model. The statistical hypothesis test is conducted using the non-parametric Kolmogorov-Smirnov test. We found evidence that shows that for low to moderate transmissibility, all empirical distribution pairs (generated from exponential and non-exponential distributions) for each of the epidemiological quantities considered are statistically dissimilar. The research in this project helps determine whether the weakening exponential distribution assumption must be considered in the estimation of probability of events defined from the empirical distribution of specific random variables. iii) The third project involves the assessment of the effect of exponentially distributed infectious periods on estimates of input parameter and the associated outcome variable predictions. Quantities unaffected by the use of exponentially distributed infectious period within low transmissibility scenarios include, the prevalence peak time, final epidemic size, epidemic duration and basic reproduction number and for high transmissibility scenarios only the prevalence peak time and final epidemic size. An application designed to determine from incidence data whether there is sufficient statistical evidence to conclude that the infectious period distribution should not be modeled by an exponential distribution is developed. A method for estimating explicitly specified non-exponential parametric probability density functions for the infectious period from epidemiological data is developed. The methodologies presented in this dissertation may be applicable to models where waiting times are used to model transitions between stages, a process that is common in the study of life-history dynamics of many ecological systems.
Understanding the Impact of Social Factors on the Transmission Dynamics of Infectious Diseases Across Highly Heterogeneous Risk Environments.
Abstract:
This dissertation explores the impact of environmental dependent risk on disease
dynamics within a Lagrangian modeling perspective; where the identity (defined by
place of residency) of individuals is preserved throughout the epidemic process. In
Chapter Three, the impact of individuals who refuse to be vaccinated is explored.
MMR vaccination and birth rate data from the State of California are used to determine
the impact of the anti-vaccine movement on the dynamics of growth of the
anti-vaccine sub-population. Dissertation results suggest that under realistic California
social dynamics scenarios, it is not possible to revert the influence of anti-vaccine
contagion. In Chapter Four, the dynamics of Zika virus are explored in two highly
distinct idealized environments defined by a parameter that models highly distinctive
levels of risk, the result of vector and host density and vector control measures. The
underlying assumption is that these two communities are intimately connected due
to economics with the impact of various patterns of mobility being incorporated via
the use of residency times. In short, a highly heterogeneous community is defined by
its risk of acquiring a Zika infection within one of two “spaces,” one lacking access to
health services or effective vector control policies (lack of resources or ignored due to
high levels of crime, or poverty, or both). Low risk regions are defined as those with
access to solid health facilities and where vector control measures are implemented
routinely. It was found that the better connected these communities are, the existence
of communities where mobility between risk regions is not hampered, lower the overall,
two patch Zika prevalence. Chapter Five focuses on the dynamics of tuberculosis
(TB), a communicable disease, also on an idealized high-low risk set up. The impact
of mobility within these two highly distinct TB-risk environments on the dynamics
and control of this disease is systematically explored. It is found that collaboration
and mobility, under some circumstances, can reduce the overall TB burden.
Computational and Analytical Mathematical Techniques for Modeling Heterogeneity
ABSTRACT
This dissertation is intended to tie together a body of work which utilizes a variety of methods to study applied mathematical models involving heterogeneity often omitted with classical modeling techniques. I posit three cogent classifications of heterogeneity: physiological, behavioral, and local (specifically connectivity in this work). I consider physiological heterogeneity using the method of transport equations to study heterogeneous susceptibility to diseases in open populations (those with births and deaths). I then present three separate models of behavioral heterogeneity. An SIS/SAS model of gonorrhea transmission in a population of highly active men-who-have-sex-with-men (MSM) is presented to study the impact of safe behavior (prevention and self-awareness) on the prevalence of this endemic disease. Behavior is modeled in this examples via static parameters describing consistent condom use and frequency of STD testing.
In an example of behavioral heterogeneity, in the absence of underlying dynamics, I present a generalization to “test theory without an answer key” (also known as cultural consensus modeling or CCM). CCM is commonly used to study the distribution of cultural knowledge within a population. The generalized framework presented allows for selecting the best model among various extensions of CCM: multiple subcultures, estimating the degree to which individuals guess yes, and making competence homogeneous in the population. This permits model selection based on the principle of information criteria. The third behaviorally heterogeneous model studies adaptive behavioral response based on epidemiological-economic theory within an SIR epidemic setting. Theorems used to analyze the stability of such models with a generalized, nonlinear incidence structure are adapted and applied to the case of standard incidence and adaptive incidence.
As an example of study in spatial heterogeneity I provide an explicit solution to a generalization of the continuous time approximation of the Albert-Barab´asi scale-free network algorithm. The solution is found by recursively solving the differential equations via integrating factors, identifying a pattern for the coefficients and then proving this observed pattern is consistent using induction. An application to disease dynamics on such evolving structures is then studied.
The Role of Environmental Context in the Dynamics and Control of Alcohol Use
ABSTRACT
Alcohol consumption is a function of social dynamics, environmental contexts, individuals’preferences and family history. Empirical surveys have not been able to rank, identify, or quantify the mechanisms responsible for heavier drinking. There have been a few attempts to apply nonlinear models to the study of the dynamics of alcohol use at the population level, but there are no systematic approaches for evaluating the impact of proposed control programs over time. In this dissertation, the role of environmental context in the dynamics of alcohol drinking is studied. First, a simple deterministic model that focuses on the impact on drinking of individuals’ traffic between low- and high-risk environments, is introduced. The model studies drinking as a socially contagious process, that is, it is assumed that “contacts” between drinkers and non-drinkers in the appropriate context, may lead to drinking. The strength of the contagion process is mediated by the drinking environment. Results shows that under certain assumptions, increases in the movement of drinkers between distinct drinking environments can increase the prevalence of heavy drinkers. However, the local culture of drinking environments as measured by the strength of social contacts within a given environment may in fact change the outcomes significantly. Model parameters from college data that include the results of a drinking survey at 14 state college campuses in California carried out by researchers at the Prevention Research Center at Berkeley, are estimated. The basic drinking reproduction number that measures the “reproductive” impact of moderate drinkers on light drinkers is derived from the model. Uncertainty and sensitivity analysis is performed to assess the variability in basic drinking reproduction number that results from error in measurement in social and environmental residence times parameters.
An extended model is then considered by assuming that random effects arise in the process of social interactions of drinkers, and increasing/decreasing of drinking levels may occur by chance mechanisms. This stochastic model is used to capture variability in drinking patterns as a result of these intrinsic naturally occurring random effects.
The effects of prevention and intervention programs on the distribution of drinking levels are investigated using the deterministic and stochastic models. Results from this systematic study of control programs suggest that prevention is better than intervention in controlling heavier drinking. However, exclusive implementation of prevention policies with perfect efficacy may not be feasible. Mutual implementation of prevention programs, and interventions in high-rsik environments is the next best option. Higher rates of intervention in low-risk environments than intervention rates in high-risk environments are also better when the aim is to control high level of drinking in the community.
Type 2 Diabetes and Obesity: A Biological, Behavioral, and Environmental Context
Abstract:
According to the CDC, type 2 diabetes accounts for 90-95% of diabetes (29.1 million) cases and manifests in 15-30% of prediabetes (86 million) cases, where 9 out of 10 individuals do not know they have prediabetes. Obesity, observed in 56.9% of diabetes cases, arises from the interactions among genetic, biological, environmental, and behavioral factors that are not well understood. Assessing the strength of these links in conjunction with the identification and evaluation of intervention strategies in vulnerable populations is central to the study of chronic diseases. This research addresses three issues that loosely connect three levels of organization utilizing a combination of quantitative and qualitative methods. First, the nonlinear dynamics between insulin, glucose, and free fatty acids is studied via a hypothesis-based model and validated with bariatric surgery data, demonstrating key metabolic factors for maintaining glucose homeostasis. Second, the challenges associated with the treatment or management, and prevention of diabetes is explored in the context of an individualized-based intervention study, highlighting the importance of diet and environment. Third, the importance of tailored school lunch programs and policies is studied through contagion models developed within a social-ecological framework. The Ratatouille Effect, motivated by a pilot study among PreK-8th grade Arizona students, is studied and exposes the importance of institutionalizing practical methods that factor in the culture, norms, and values of the community. The outcomes of this research illustrate an integrative framework that bridges physiological, individual, and population level approaches to study type 2 diabetes and obesity from a holistic perspective. This work reveals the significance of utilizing observational and experimental studies merged with the application of quantitative and qualitative methods to better elucidate underlying causes of chronic diseases and for developing solutions that lead to sustainable healthy behaviors, and more importantly, the need for translatable multilevel methodologies for the study of the progression, treatment, and prevention of chronic diseases from a multidisciplinary perspective.
Cities in Ecology: Settlement Patterns and Diseases
ABSTRACT
A sequence of models is developed to describe urban population growth in the context of the embedded physical, social and economic environments and an urban disease are developed. This set of models is focused on urban growth and the relationship between the desire to move and the utility derived from city life. This utility is measured in terms of the economic opportunities in the city, the level of human constructed amenity, and the level of amenity caused by the natural environment. The set of urban disease models is focused on examining prospects of eliminating a disease for which a vaccine does not exist. It is inspired by an outbreak of the vector-borne disease dengue fever in Peru, during 2000-2001.
A mathematical study on the emergence of collective differences from individual variation: The complex adaptive dynamics of eusocial-insect colonies
ABSTRACT
Variation in living systems and how it cascades across organizational levels is central to biology. To understand the constraints and amplifications of variation in collective systems, I mathematically study how group-level differences emerge from individual variation in eusocial-insect colonies, which are inherently diverse and easily observable individually and collectively. Considering collective processes in three species where increasing degrees of heterogeneity are relevant, I address how individual variation scales to colony-level variation and to what degree it is adaptive. First, I introduce a Markov-chain decision model for stochastic individual quorum-based recruitment decisions of rock-ant workers during house hunting, and how they determine collective speed--accuracy balance. Differences in the average threshold-dependent response characteristics of workers between colonies cause collective differences in decision-making. Moreover, noisy behavior may prevent drastic collective cascading into poor nests. Next, I develop an ODE model to study how cognitive diversity among honey-bee foragers influences collective attention allocation between novel and familiar resources. Results provide a mechanistic basis for changes in foraging activity and preference with group composition. Moreover, sensitivity analysis reveals that the main individual driver for foraging allocation shifts from recruitment (communication) to persistence (independent effort) as colony composition changes. This might favor specific degrees of heterogeneity that best amplify communication in wild colonies. Lastly, I consider diversity in size, age, and task for nest defense in stingless bees. To better understand how these dimensions of diversity interact to balance defensive demands with other colony needs, I study their effect on colony size and task allocation through a demographic Filippov ODE model. Along each dimension, variation is beneficial in a certain range, outside of which colony adaptation and survival are compromised. This work elucidates how variation in collective properties emerges from nonlinear interactions between varying components in eusocial insects, but it can be generalized to other biological systems with similar fundamental characteristics but less empirical tractability. Moreover, it has the potential of inspiring algorithms that capitalize on heterogeneity in engineered systems where simple components with limited information and no central control must solve complex tasks.
Mathematical Model for IL-6-Mediated Tumor Growth, and Targeted Treatment
Abstract:
Head and neck squamous cell carcinoma (HNSCC), the sixth most common cancer type worldwide, accounts for more than 630,000 new cases and 350,000 deaths annually. Drug-resistance and tumor recurrence are the most challenging problems in head and neck cancer treatment. It is hypothesized that a very small fraction of stem-like cells within HNSCC tumor, called cancer stem cells (CSCs), is responsible for tumor initiation, progression, resistance and recurrence. It has also been shown that IL-6 secreted by head and neck tumor-associated endothelial cells (ECs) enhances the survival, self-renewal and tumorigenic potential of head and neck CSCs. In this study we will use a mathematical multi-scale model which operates at the intracellular, molecular, and tissue level to investigate the impacts of EC-secreted IL-6 signaling on the crosstalk between tumor cells and ECs during tumor growth. This model will be calibrated by using the experimental in vivo data. Eventually the model will be modified to explore the responses of head and neck cancer cells to combination therapy involving Tocilizumab (an anti-IL-6R anti-body) and Cisplatin (the most frequently used chemotherapy for head and neck cancer). The model will be able to predict the final proportion of CSCs in response to endothelial cell-secreted IL-6 and drug therapies. The model will be validated by directly comparing the experimental treatment data and the model predictions. This could potentially provide a condition under which we could control enlargement of the head and neck CSC pool and tumor recurrence. It may also suggest the best bounds for Cisplatin and/or Tocilizumab dose and frequency to be tested in the clinical trial.
Modeling the Transmission of Vancomyncin-Resistant Enterococcus in Hospitals: A Case Study
ABSTRACT
Nosocomial, or hospital-acquired infections, the fourth cause of death in the US, means that hospitals provide not only medical care but also harbor pathogens that pose serious risks of infection, often fatal, particularly to the young, the elderly, and immune-compromised individuals. Infection-control measures aimed at reducing their impact are being implemented with various degrees of efficiency at US hospitals. Vancomycin-resistant Enterococcus (VRE), one of the most prevalent and dangerous pathogens involved, general and oncology hospital units data are used to highlight the importance of modeling nosocomial infection dynamics as a prelude to the testing and evaluation of control measures.
New mathematical models of the transmission dynamics of VRE in hospitals are introduced in order to identify and quantitatively assess the time evolution of nosocomial infections. Ordinary differential equation (ODE) and discrete delay differential (DDD) models in conjunction with statistical methods are used to estimate key population-level nosocomial transmission parameters. This framework is tested using unpublished surveillance data from two types of hospital units. The population is divided into uncolonized, VRE colonized, and VRE colonized-in-isolation categories and the use of constant and variable rates of isolation admitted with VRE or VRE-colonized during hospital stays is evaluated in models that account for health care workers’ hand-hygiene compliance. The process of model calibration detected irregularities in the available surveillance data, irregularities that are most likely the result of the data recording-process. Efforts to fit data within our highly flexible dynamic-modeling framework turned out to require a clinical-trial level surveillance data.
The usefulness flexible and general new modeling framework of the transmission dynamics of nosocomial infections like VRE was first evaluated using synthetic noisy data and then tested against the available data. Parameters whose estimates we need for the testing and evaluation competing or integrated intervention and control measures via mathematical models could not be accurately computed from available data. We conclude that it would be extremely difficult take advantage of transmission dynamic models in the fight against nosocomial infections unless systemic and highly controlled efforts to collect data are put in place at hospitals.
Modeling the Role of Land-Use Change on the Spread of Infectious Disease
ABSTRACT
Land-use change has arguably been the largest contributor to the emergence of novel zoonotic diseases within the past century. However, the relationship between patterns of land-use change and the resulting landscape configuration on disease spread are poorly understood as current cross-species disease transmission models have not adequately incorporated spatial features of habitats. Furthermore, mathematical-epidemiological studies have not considered the role that land-use change plays in disease transmission throughout an ecosystem.
This dissertation models how a landscape's configuration, examining the amount and shape of habitat overlap, contributes to cross-species disease transmission to determine the role that land-use change has on the spread of infectious diseases. To approach this, an epidemiological model of transmission between a domesticated and a wild species is constructed. Each species is homogeneously mixed in its respective habitat and heterogeneously mixed in the habitat overlap, where cross-species transmission occurs. Habitat overlap is modeled using landscape ecology metrics.
This general framework is then applied to brucellosis transmission between elk and cattle in the Greater Yellowstone Ecosystem. The application of the general framework allows for the exploration of how land-use change has contributed to brucellosis prevalence in these two species, and how land management can be utilized to control disease transmission. This model is then extended to include a third species, bison, in order to provide insight to the indirect consequences of disease transmission for a species that is situated on land that has not been converted. The results of this study can ultimately help stakeholders develop policy for controlling brucellosis transmission between livestock, elk, and bison, and in turn, could lead to less disease prevalence, reduce associated costs, and assist in population management.
This research contributes novelty by combining landscape ecology metrics with theoretical epidemiological models to understand how the shape, size, and distribution of habitat fragments on a landscape affect cross-species disease transmission. The general framework demonstrates how habitat edge in single patch impacts cross-species disease transmission. The application to brucellosis transmission in the Greater Yellowstone Ecosystem between elk, cattle, and bison is original research that enhances understanding of how land conversion is associated with enzootic disease spread.
On the Dynamics of Infectious Diseases in Modern Landscapes: Urban Settings and Drug Resistance
ABSTRACT
Extraordinary medical advances have led to significant reductions in the burden of infectious diseases in humans. However, infectious diseases still account for more than 13 million annual deaths. This large burden is partly due to some pathogens having found suitable conditions to emerge and spread in denser and more connected host populations, and others having evolved to escape the pressures imposed by the rampant use of antibiotics. It is then critical to improve our understanding of how diseases spread in these modern landscapes, characterized by new host population structures and socio-economic environments, as well as containment measures such as the deployment of drugs. Thus, the motivation of this dissertation is two-fold. First, we study, using both data-driven and modeling approaches, the the spread of infectious diseases in urban areas. As a case study, we use confirmed-cases data on sexually transmitted diseases (STDs) in the United States to assess the conduciveness of population size of urban areas and their socio-economic characteristics as predictors of STD incidence. We find that the scaling of STD incidence in cities is superlinear, and that the percent of African-Americans residing in cities largely determines these statistical patterns. Since disparities in access to health care are often exacerbated in urban areas, within this project we also develop two modeling frameworks to study the effect of health care disparities on epidemic outcomes.
Discrepant results between the two approaches indicate that knowledge of the shape of the recovery period distribution, not just its mean and variance, is key for assessing the epidemiological impact of inequalities.
The second project proposes to study, from a modeling perspective, the spread of drug resistance in human populations featuring vital dynamics, stochasticity and contact structure. We derive effective treatment regimes that minimize both the overall disease burden and the spread of resistance. Additionally, targeted treatment in structured host populations may lead to higher levels of drug resistance, and if drug-resistant strains are compensated, they can spread widely even when the wild-type strain is below its epidemic threshold.
Behavioral Kinetics: Interrogating Canonical Models in Population Dynamics
ABSTRACT
There is a need in the ecology literature to have a discussion about the fundamental theories from which population dynamics arises. An ad hoc approach to model development has gripped the field; this must be replaced with hard thinking. The phenomena of population growth can be captured by almost any sigmoid function. The question of which sigmoid function best explains a data set cannot be answered meaningfully by statistical regression since that can only speak to the validity of the shape. There is a need to revisit enzyme kinetics and ecological stoichiometry to properly justify basal model selection in ecology.
This dissertation derives several common population growth models from a generalized equation. The mechanistic validity of these models in different contexts is explored through a kinetic lens. The behavioral kinetic framework is then put to the test by examining a set of biologically plausible growth models against the 1968-1995 elk population count data for northern Yellowstone. Using only this count data, the novel Monod-Holling growth model was able to accurately predict minimum viable population and life expectancy despite both being exogenous to the model and data set. Lastly, the elk/wolf data from Yellowstone was used to compare the validity of the Rosenzweig-MacArthur and Arditi-Ginzburg models. They both were derived from a more general model which included both predator and prey mediated steps. The Arditi-Ginzburg model was able to fit the training data better, but only the Rosenzweig-MacArthur model matched the validation data.
Accounting for animal sexual behavior allowed for the creation of the Monod-Holling model which is just as simple as the logistic differential equation but provides greater insights for conservation purposes. Explicitly acknowledging the ethology of wolf predation helps explain the differences in predictive performances by the best fit Rosenzweig-MacArthur and Arditi-Ginzburg models. The behavioral kinetic framework has proven to be a useful tool, and it has the ability to provide even further insights going forward.
Modeling Transient and Sustained Epidemic Dynamics: Cholera, Influenza and Rubella as Case Studies
ABSTRACT
Increasing our understanding of the underlying mechanisms driving epidemics has public health value for the control of infectious diseases. Mathematical models can be useful to quantify specific aspects of the transmission dynamics of infectious diseases. The present work focuses on the development of techniques to model transient and sustained epidemiological outbreak patterns that are characteristic of diseases such as cholera, influenza and rubella. First, we develop a stochastic model describing the interactions between humans and an aquatic reservoir contaminated with V. cholerae in an effort to reproduce sustained oscillations observed in epidemiological data. We show that in a limiting sense, the stochastic path of our system, as observed in simulations, approximates a circular motion modulated by an Ornstein-Uhlenbeck process. Our results allow us to explore appropriate parameter regimes where the phenomenon of sustained oscillations can be observed. Secondly, we also examine the transient setting of historical pandemic influenza as observed during the 1918 influenza pandemic and use pandemic data from Geneva, Switzerland. Here, we model the transmission dynamics of two strains of influenza interacting via cross-immunity to simulate two temporal waves of influenza and explore the impact of the basic reproduction number, as a measure of transmissibility associated to each influenza strain, cross-immunity and the timing of the onset of the second influenza variant on the pandemic profile. Our results indicate that avoiding a second influenza infection is plausible given sufficient levels of cross-protection are attained via natural infection during an early wave of infection or vaccination campaigns prior to a second wave. Lastly, we examine the patterns of rubella transmission using data from Peru's national epidemiological surveillance system to assess the effects of intervention strategies such as vaccination. For this purpose we use highly refined spatial, temporal and age-specific incidence data of Peru, a geographically diverse country, to quantify spatial-temporal patterns of incidence and transmissibility for rubella during the period 1997-2006. These findings highlight the importance of incorporating the space dimension and in appropriately disentangling and interpreting the relevant patterns of seasonality and persistence of infectious diseases.
Assessing the Effects of Institutional and Spatial Arrangements in Analytical and Computational Models of Conservation
ABSTRACT
This work is an assemblage of three applied projects that address the institutional and spatial constraints to managing threatened and endangered (T & E) terrestrial species. The first project looks at the role of the Endangered Species Act (ESA) in protecting wildlife and whether banning non-conservation activities on multi-use federal lands is socially optimal. A bioeconomic model is used to identify scenarios where ESA-imposed regulations emerge as optimal strategies and to facilitate discussion on feasible long-term strategies in light of the ongoing public land-use debate. Results suggest that banning harmful activities is a preferred strategy when valued species are in decline or exposed to poor habitat quality. However such a strategy cannot be sustained in perpetuity, a switch to land-use practices characteristic of habitat conservation plans is recommended. The spatial portion of this study is motivated by the need for a more systematic quantification and assessment of landscape structure ahead of species reintroduction; this portion is further broken up into two parts. The first explores how connectivity between habitat patches promotes coexistence among multiple interacting species. An agent-based model of a two-patch metapopulation is developed with local predator-prey dynamics and density-dependent dispersal. The simulation experiment suggests that connectivity levels at both extremes, representing very little risk and high risk of species mortality, do not augment the likelihood of coexistence while intermediate levels do. Furthermore, the probability of coexistence increases and spans a wide range of connectivity levels when individual dispersal is less probabilistic and more dependent on population feedback. Second, a novel approach to quantifying network structure is developed using the statistical method of moments. This measurement framework is then used to index habitat networks and assess their capacity to drive three main ecological processes: dispersal, survival, and coexistence. Results indicate that the moments approach outperforms single summary metrics and accounts for a majority of the variation in process outcomes. The hierarchical measurement scheme is helpful for indicating when additional structural information is needed to determine ecological function. However, the qualitative trend between network indicator and function is, at times, unintuitive and unstable in certain areas of the metric space.
Biophysical Mechanism for Neural Spiking Dynamics:
ABSTRACT:
In the honey bee antennal lobe, uniglomerular projection neurons (uPNs) transiently spike to odour sensory stimuli with odour-specific response latencies, i.e., delays to first spike after odour stimulation onset. Recent calcium imaging studies show that the spatio-temporal response profile of the activated uPNs are dynamic and changes as a result of associative conditioning, facilitating odour-detection of learned odours. Moreover, odour-representation in the antennal lobe undergo reward-mediated plasticity processes that increase response delay variations in the activated ensemble of uniglomerular projection neurons. Octopamine is necessarily involved in these plasticity processes. Yet, the cellular mechanisms are not well understood. I hypothesize that octopamine modulates cholinergic transmission to uPNs by triggering translation and upregulation of nicotinic receptors, which are more permeable to calcium. Consequently, this increased calcium-influx signals transcription factors that upregulate potassium channels in the dendritic cortex of glomeruli, similar to synaptic plasticity mechanisms recently shown in various insect species. A biophysical model of the antennal lobe circuit is developed in order to test the hypothesis that increased potassium channel expression in uPNs mediate response delays to first spike, dynamically tuning odour-representations to facilitate odour-detection of learned odours.
Immune Response in the Study of Infectious Diseases (Co-Infection) in an Endemic Region
ABSTRACT
Diseases have been part of human life for generations and evolve within the population, sometimes dying out while other times becoming endemic or the cause of recurrent outbreaks. The long term influence of a disease stems from dierent dynamics within or between pathogen-host, that have been analyzed and studied by many researchers using mathematical models. Co-infection with dierent pathogens is common, yet little is known about how infection with one pathogen aects the host's immunological response to another. Moreover, no work has been found in the literature that considers the variability of the host immune health or that examines a disease at the population level and its corresponding interconnectedness with the host immune system.
Knowing that the spread of the disease in the population starts at the individual level, this thesis explores how variability in immune system response within an endemic environment aeffects an individual's vulnerability, and how prone it is to co-infections. Immunology-based models of Malaria and Tuberculosis (TB) are constructed by extending and modifying existing mathematical models in the literature. The two are then combined to give a single nine-variable model of co-infection with Malaria and TB. Because these models are dicult to gain any insight analytically due to the large number of parameters, a phenomenological model of co-infection is proposed with subsystems corresponding to the individual immunology-based model of a single infection. Within this phenomenological model, the variability of the host immune health is also incorporated through three different pathogen response curves using nonlinear bounded Michaelis-Menten functions that describe the level or state of immune system (healthy, moderate and severely compromised). The immunology-based models of Malaria and TB give numerical results that agree with the biological observations. The Malaria{TB co-infection model gives reasonable results and these suggest that the order in which the two diseases are introduced have an impact on the behavior of both. The subsystems of the phenomenological models that correspond to a single infection (either of Malaria or TB) mimic much of the observed behavior of the immunology-based counterpart and can demonstrate different behavior depending on the chosen pathogen response curve. In addition, varying some of the parameters and initial conditions in the phenomenological model yields a range of topologically different mathematical behaviors, which suggests that this behavior may be able to be observed in the immunology-based models as well.
The phenomenological models clearly replicate the qualitative behavior of primary and secondary infection as well as co-infection. The mathematical solutions of the models correspond to the fundamental states described by immunologists: virgin state, immune state and tolerance state. The phenomenological model of co-infection also demonstrates a range of parameter values and initial conditions in which the introduction of a second disease causes both diseases to grow without bound even though those same parameters and initial conditions did not yield unbounded growth in the corresponding subsystems. This results applies to all three states of the host immune system. In terms of the immunology-based system, this would suggest the following: there may be parameter values and initial conditions in which a person can clear Malaria or TB (separately) from their system but in which the presence of both can result in the person dying of one of the diseases. Finally, this thesis studies links between epidemiology (population level) and immunology in an effort to assess the impact of pathogen's spread within the population on the immune response of individuals. Models of Malaria and TB are proposed that incorporate the immune system of the host into a mathematical model of an epidemic at the population level.
Oncolytic Viral and Immunotherapy Models Combined with Strategies to Ameliorate Cancer Burden
Abstract:
Combination therapy has shown to improve success for cancer treat-
ment. Oncolytic virotherapy is cancer treatment that uses engineered
viruses to speci_cally infect and kill cancer cells, without harming healthy
cells. Immunotherapy boosts the body's natural defenses towards can-
cer. The combination of oncolytic virotherapy and immunotherapy is ex-
plored through deterministic systems of nonlinear di_erential equations,
constructed to match experimental data for murine melanoma. Math-
ematical analysis was done in order to gain insight on the relationship
between cancer, viruses and immune response. The model was extended
to serve towards clinical needs, with the underlying goal to seek optimal
treatment regimens; for both frequency and dose quantity. The models in
this work were _rst used to estimate parameters from preclinical exper-
imental data, to identify biologically realistic parameter values. Insight
gained from the mathematical analysis in the _rst model, allowed for nu-
merical analysis to explore optimal treatment regimens of combination
oncolytic virotherapy and dendritic vaccinations. Permutations account-
ing for treatment scheduled were done to _nd regimens that reduce tumor
size. Observations from the produced data lead to in silico exploration of
immune-viral interactions. Results suggest under optimal settings, com-
bination treatment works better than monotherapy of either type. The
most optimal result indicates administering treatment over a longer period
of time, with fractioned doses, while reducing the total dendritic vacci-
nation quantity, and maintaining the maximum virotherapy used in the
experimental work.
A Mathematical Model of Dopamine Neurotransmission
Abstract
Dopamine (DA) is a neurotransmitter involved in attention, goal oriented behavior, movement, reward learning, and short term and working memory. For the past four decades, mathematical and computational modeling approaches have been useful in DA research, and although every modeling approach has limitations, a model is an efficient way to generate and explore hypotheses. This work develops a model of DA dynamics in a representative, single DA neuron by integrating previous experimental, theoretical and computational research. The model consists of three compartments: the cytosol, the vesicles, and the extracellular space and forms the basis of a new mathematical paradigm for examining the dynamics of DA synthesis, storage, release and reuptake. The model can be driven by action potentials generated by any model of excitable membrane potential or even from experimentally induced depolarization voltage recordings. Here the model is forced by a previously published model of the excitable membrane of a mesencephalic DA neuron in order to study the biochemical processes involved in extracellular DA production. After demonstrating that the model exhibits realistic dynamics resembling those observed experimentally, the model is used to examine the functional changes in presynaptic mechanisms due to application of cocaine. Sensitivity analysis and numerical studies that focus on various possible mechanisms for the inhibition of DAT by cocaine provide insight for the complex interactions involved in DA dynamics. In particular, comparing numerical results for a mixed inhibition mechanism to those for competitive, non-competitive and uncompetitive inhibition mechanisms reveals many behavioral similarities for these different types of inhibition that depend on inhibition parameters and levels of cocaine. Placing experimental results within this context of mixed inhibition provides a possible explanation for the conflicting views of uptake inhibition mechanisms found in experimental neuroscience literature.
Mathematical Modeling of Systematic Treatment Implementation and Dynamics of Neglected Tropical Diseases: Case Studies of Visceral Leishmaniasis & Soil-Transmitted Helminths
ABSTRACT
Neglected tropical diseases (NTDs) comprise of diverse communicable diseases that affect mostly the developing economies of the world, the “neglected” populations. The NTDs Visceral Leishmaniasis (VL) and Soil-transmitted Helminthiasis (STH) are among the top contributors of global mortality and/or morbidity. They affect resource-limited regions (poor health-care literacy, infrastructure, etc.) and patients’ treatment behavior is irregular due to the social constraints. Through two case studies, VL in India and STH in Ghana, this work aims to: (i) identify the additional and potential hidden high-risk population and its behaviors critical for improvinginterventions and surveillance; (ii) develop models with those behaviors to study therole of improved control programs on diseases’ dynamics; (iii) optimize resources for treatment-related interventions. Treatment non-adherence is a less focused (so far) but crucial factor for the hindrance in WHO’s past VL elimination goals. Moreover, treatment non-adherers, hidden from surveillance, lead to high case-underreporting. Dynamical models are developed capturing the role of treatment-related human behaviors (patients’ infectivity, treatment access and non-adherence) on VL dynamics. The results suggest that the average duration of treatment adherence must be increased from currently 10 days to 17 days for a 28-day Miltefosine treatment to eliminate VL. For STH, children are considered as a high-risk group due to theirhygiene behaviors leading to higher exposure to contamination. Hence, Ghana, a resource-limited country, currently implements a school-based Mass Drug Administration (sMDA) program only among children. School staff (adults), equally exposed to this high environmental contamination of STH, are largely ignored under the current MDA program. Cost-effective MDA policies were modeled and compared using alternative definitions of “high-risk population”. This work optimized and evaluated how MDA along with the treatment for high-risk adults makes a significant improvement in STH control under the same budget. The criticality of risk-structured modeling depends on the infectivity coefficient being substantially different for the two adult risk groups. This dissertation pioneers in highlighting the cruciality of treatment-related risk groups for NTD-control. It provides novel approaches to quantify relevant metrics and impact of population factors. Compliance with the principles and strategies from this study would require a change in political thinking in the neglected regions in order to achieve persistent NTD-control.
Deterministic and Stochastic Metapopulation Models for Dengue Fever
ABSTRACT
A spatial temporal data set of dengue in Peru from 1994-2008 was made available to us by the Ministry of Health of Peru and the analyses of its spatio-temporal patterns motivated the work in this dissertation. We found that aggregated reported data masked the spatio-temporal patterns of dengue over this window in time. A series of models are presented in this dissertation in order to identify mechanisms that capture observed patterns. We have in fact identified a framework capable of capturing dengue outbreaks in Peru. Deterministic and stochastic single and two-patch models are introduced and some of their properties identified via some mathematical analyses complemented with extensive simulations. We find that the asymptotics of the mean field model (final size epidemic), while useful, mask critical details that are central to control and public health policies. We introduce a stochastic migration model that allows us to construct a family of distributions of time T , which the first infected individual leaves the “home” patch, and estimate the variance along the CDF. A two patch model, where each person has a positive probability of being in a patch alternate to his home location, shows the effect coupling coefficients will have on the time between the epidemic peaks. The inclusion of seasonality and human demographics to the two patch model leads to reoccurring outbreaks, as seen in the data. The two approaches of modeling migration, though different mathematically, complement each other in explaining what factors affect the spread of dengue. Finally, optimal control methods are incorporated into our models. We consider what strategy should be used if the objective is to minimize the total number of infected individuals, at a minimal cost, during a fixed time interval. When control is applied to a patch with the basic reproductive number R0 below a certain threshold, it effectively stops the epidemic. Also, control will delay the spread of dengue from one patch to another.
Size Structured Epidemic Models
ABSTRACT
There have been many studies on the dynamics of infectious diseases considering the age structure of the population. This study analyzes the dynamics when the population is stratified by size. This kind of models are useful in the spread of a disease in fisheries where size matters, for microorganism populations or even human diseases that are driven by weight.
A simple size-structured SIR model is introduced for which a threshold condition, R0, equilibria and stability are established in special cases.
Hethcote's approach is used to derive, from first principles, a parallel ODE size-structure system involving $n$-size classes.The specific case of n=2 is partially analyzed. Constant effort harvesting is added to this model with the purpose of exploring the role of controls and harvesting. Different harvesting policies are proposed and analyzed through simulations.
Statistical Signal Processing for Graphs
ABSTRACT
Analysis of social networks has the potential to provide insights into wide range of applications. As datasets continue to grow, a key challenge is the lack of a widely applicable algorithmic framework for detection of statistically anomalous networks and network properties. Unlike traditional signal processing, where models of truth or empirical verification and background data exist and are often well defined, these features are commonly lacking in social and other networks. Here, a novel algorithmic framework for statistical signal processing for graphs is presented. The framework is based on the analysis of spectral properties of the residuals matrix. The framework is applied to the detection of innovation patterns in publication networks, leveraging well-studied empirical knowledge from the history of science. Both the framework itself and the application constitute novel contributions, while advancing algorithmic and mathematical techniques for graph-based data and understanding of the patterns of emergence of novel scientific research. Results indicate the efficacy of the approach and highlight a number of fruitful future directions.
A Mathematical study of Mechanisms and Consequences of task Organization in Animal Group
Abstract:
The question of how groups collectively organize around work is fundamental to our understanding of cooperation in animal societies. Mathematical models have been instrumental in unraveling how group-level patterns of task organization can spontaneously develop from inter-individual differences based on the concept of behavioral response-thresholds. However, these models have rarely focused on the individual-level mechanisms generating response thresholds or examined their consequences. My work argues for a more principled empirically driven approach that starts with a theory of how individuals make behavioral decisions in costly environments. Armed with this information, we can construct mathematical models that can be applied in a broader range of contexts where animals cooperate.
First, I discuss a modeling study we developed to understand how desert harvester ant colonies regulate their foraging activity in response to changes environmental profitability. Theoretical results show that a key part of this regulation relates to how uncommitted foragers adapt their behavior to local information from success foragers. Moreover, this dynamic might explain why colonies vary in how robust they are to disturbances, and how fast they tend to recover from them. Next, I discuss an experimental study we developed to understand patterns of division of labor (DOL) and productivity in incipient foundress associations (groups of unrelated ant queens during colony founding stage). Results show that although scaled with group size, it had mixed per-capita effects on offspring produced. An agent-based model of the system suggest that this pattern may have developed in part due to how queens weigh costs of activity in small versus large groups. Finally, I will propose a theoretical multi-agent framework that can be used to study the dynamics of task choice and organization in social groups. The model is inspired by replicator game dynamics, and is broadly developed to include factors that affect individual behavior such as: internal constraints, task preferences, experience (i.e., self-reinforcement learning), and social facilitation.
Theoretical Studies on a Two Strain Model of Drug Resistance: Understand, Predict and Control the Emergence of Drug Resistance
ABSTRACT
Infectious diseases are a leading cause of death worldwide. With the development of drugs, vaccines and antibiotics, it was believed that for the rst time in human history diseases would no longer be a major cause of mortality. Newly emerging diseases, re-emerging diseases and the emergence of microorganisms resistant to existing treatment have forced us to re-evaluate our optimistic perspective. In this study, a simple mathematical framework for super-infection is considered in order to explore the transmission dynamics of drug-resistance. Through its theoretical analysis, we identify the conditions necessary for the coexistence between sensitive strains and drug-resistant strains. Further, in order to investigate the eectiveness of control measures, the model is extended so as to include vaccination and treatment. The impact that these pre- ventive and control measures may have on its disease dynamics is evaluated. Theoretical results being conrmed via numerical simulations.
Our theoretical results on two-strain drug-resistance models are applied in the context of Malaria, antimalarial drugs, and the administration of a possible partially eective vaccine. The objective is to develop a monitoring epidemiological framework that help evaluate the impact of antimalarial drugs and partially-eective vaccine in reducing the disease burden at the population level.
Optimal control theory is applied in the context of this framework in order to assess the impact of time dependent cost-eective treatment eorts. It is shown that cost-eective com- binations of treatment eorts depend on the population size, cost of implementing treatment controls, and the parameters of the model. We use these results to identify optimal control strategies for several scenarios.
Solution Methods for Certain Evolution Equations
ABSTRACT
Solution methods for certain linear and nonlinear evolution equations are presented in this dissertation. Emphasis is placed mainly on the analytical treatment of nonautonomous differential equations, which are challenging to solve despite the existent numerical and symbolic computational software programs available. Ideas from the transformation theory are adopted allowing one to solve the problems under consideration from a non-traditional perspective.
First, the Cauchy initial value problem is considered for a class of nonautonomous and inhomogeneous linear diffusion-type equation on the entire real line. Explicit transformations are used to reduce the equations under study to their corresponding standard forms emphasizing on natural relations with certain Riccati(and/or Ermakov)-type systems. These relations give solvability results for the Cauchy problem of the parabolic equation considered. The superposition principle allows to solve formally this problem from an unconventional point of view. An eigenfunction expansion approach is also considered for this general evolution equation. Examples considered to corroborate the efficacy of the proposed solution methods include the Fokker-Planck equation, the Black-Scholes model and the one-factor Gaussian Hull-White model.
The results obtained in the first part are used to solve the Cauchy initial value problem for certain inhomogeneous Burgers-type equation. The connection between linear (the Diffusion-type) and nonlinear (Burgers-type) parabolic equations is stress in order to establish a strong commutative relation. Traveling wave solutions of a nonautonomous Burgers equation are also investigated.
Finally, it is constructed explicitly the minimum-uncertainty squeezed states for quantum harmonic oscillators. They are derived by the action of corresponding maximal kinematical invariance group on the standard ground state solution. It is shown that the product of the variances attains the required minimum value only at the instances that one variance is a minimum and the other is a maximum, when the squeezing of one of the variances occurs. Such explicit construction is possible due to the relation between the diffusion-type equation studied in the first part and the time-dependent Schrodinger equation. A modification of the radiation field operators for squeezed photons in a perfect cavity is also suggested with the help of a nonstandard solution of Heisenberg's equation of motion.
Mathematical Modeling of Infectious Diseases
ABSTRACT
The current coronavirus disease 2019 (COVID-19) pandemic has highlighted the crucial role of mathematical models in predicting, assessing, and controlling potential outbreaks. Numerous modeling studies using statistics or differential equations have been proposed to analyze the COVID-19 dynamics, with network analysis and cluster analysis also being adapted to understand disease transmission from multiple perspectives. This dissertation explores the use of network science and mathematical models to improve the understanding of infectious diseases. Chapter 1 provides an introduction to infectious disease modeling, its history, importance, and challenges. It also introduces network science as a powerful tool for understanding the complex interactions between individuals that can facilitate disease spread. Chapter 2 develops a statistical model that describes HIV infection and disease progression in an men who have sex with men cohort in Japan receiving a PreExposure Prophylaxis (PrEP) program. The cost-effectiveness of the PrEP program was evaluated by comparing the incremental cost-effectiveness ratio over a 30-year period against the willingness to pay threshold. Chapter 3 presents an ordinary differential equations model to describe disease transmission and the effects of vaccination and mobility restrictions. Chapter 4 extends the ODE model to include spatial heterogeneity and presents partial differential equations models. These models describe the combined effects of local transmission, transboundary transmission, and human intervention on COVID19 dynamics. Finally, Chapter 5 concludes the dissertation by emphasizing the importance of developing relevant disease models to understand and predict the spread of infectious diseases by combining network science and mathematical tools.
ABSTRACT
Interactions among individuals and entities shape the dynamics of systems across various domains, from ecological populations to human societies and human-automation interfaces. In this dissertation, I investigate cooperation behavior and its implications at different levels of goal complexity within diverse types of interactions.
First, I delve into the exploration of human-automation interaction by investigating the dynamics of trust in autonomous systems. By examining key elements that influence human trust and decision-making in a changing environment, I illuminate how trust influences collaboration and performance in human-autonomy teams. Lastly, I propose a dynamic modeling framework to measure human trust in automation.
Transitioning to human-human interaction, I investigate communication dynamics within teams and their impact on performance. Through a mathematical modeling framework, I explore how effective communication fosters cooperation among team members, enabling them to achieve shared goals in diverse task environments. This project underlines the importance of communication in navigating and completing complex team tasks.
The subsequent Chapter 4 delves into human-nature interaction, exploring natural resource exploitation and its preservation for cancer treatment. Through mathematical modeling, I investigate strategies to sustainably manage natural resources while meeting public health needs. This highlights the complexity of balancing human interests with the preservation of the ecosystem.
In the domain of inter-species interaction, Chapter 5 examines the cooperative behaviors within ant colonies, where ants collaborate to achieve collective goals such as successful nest growth and founding. By studying the dynamics of different nest founding strategies from queen ants, I investigate how cooperation facilitates complex tasks and influences colony survival, shedding light on the adaptive significance of social organization in insects.
By combining findings across these projects, this dissertation offers insights into the fundamental aspects of cooperation and goal complexity in different interactive contexts. It contributes to my understanding of how cooperation impacts relationships and dynamics from an individual level to a group level in domains ranging from ecology to human teams. These findings inform strategies for advancing and strengthening collaboration in complex environments.
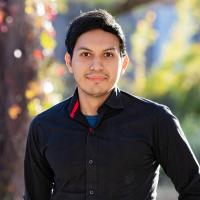
Mathematical and Computational Modeling of Collective Dynamics
ABSTRACT
Collectives are fundamental units of collaboration that range from dyads to large groups, encompassing diverse compositions such as all-human assemblies, human-automation collaborations, and even natural collectives. Understanding the complex interactions that drive collective behavior is essential for enhancing performance, resilience, and adaptability across various contexts. This dissertation employs mathematical and computational modeling to investigate collective dynamics through three interconnected projects.
The first project examines human-automation collaborations by analyzing how individuals rely on automated systems. It identifies the key factors that influence automation usage and develops models to predict automation usage in real-time. These insights aim to enhance safety and optimize collaboration between humans and automation in dynamic environments. The second project focuses on the dynamics of communication within dyad teams. Utilizing continuous-time dynamical models, this research uncovers communication patterns that improve team performance and effectiveness. The findings provide valuable guidelines for designing more responsive and efficient autonomous systems. The third project investigates honeybee colonies as adaptive biological collectives. By integrating temperature-dependent growth rates into population models, this study reveals how environmental factors like temperature impact the dynamics and stability of honeybee populations. These results offer important implications for ecological management and conservation efforts.
Collectively, these projects deepen the understanding of how individual behaviors and environmental conditions shape the effectiveness and stability of collectives. The integrated findings inform the development of strategies to improve collaboration and sustainability in both engineered and natural systems, contributing to advancements in human factors engineering, adaptive system design, and ecology.
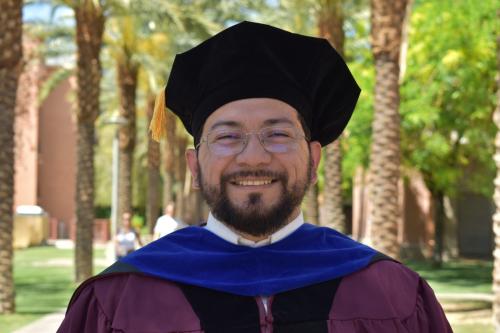
Quantifying the Impact of Partner Selection on Viral Sexually Transmitted Infections in the United States
ABSTRACT
A compartmental mathematical model has been developed to analyze the impact of partner selection on the transmission dynamics of viral sexually transmitted infections (STIs), with a particular focus on Human Immunodeficiency Virus (HIV). A slightly modified second model is then applied to study Human Papillomavirus (HPV). These models consider a population of men and women who may engage in sexual contact with partners of the opposite sex, same sex, or both. Later, to isolate the effects of partner choice, a multigroup approach is proposed, separating the population into subgroups based on partner preferences. This study examines how partner selection patterns influence STI transmission dynamics, comparing populations that engage exclusively in opposite-sex interactions with those involved in non-opposite-sex interactions. Using a multigroup modeling approach, we assess the influence of recruitment rates, initial population distributions, and dominant transmission pathways on epidemic dynamics. A key objective is determining whether increasing female vaccination coverage alone can significantly reduce HPV transmission, as expected under a universal vaccination program. The findings point to the crucial role of bisexual subgroups in sustaining transmission and demonstrate how variations in recruitment among subgroups shape epidemic outcomes. These insights provide a foundation for public health strategies aimed at mitigating STI spread, reducing HPV incidence, and preventing HPV-related cancers. Sensitivity analysis reveals that female vaccination coverage has the most significant impact on reducing the control reproduction number followed by vaccine efficacy and male vaccination coverage.